Top 10: Machine Learning Innovations
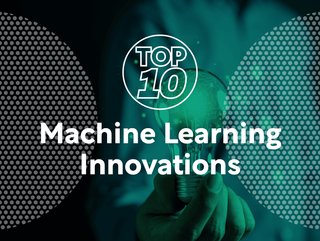
Machine learning is vastly changing the business landscape, as organisations are keen to harness it to improve their outlook.
IBM describes machine learning as a branch of artificial intelligence (AI) and computer science that focuses on the use of data and algorithms to imitate the way that humans learn. As a result, it is able to gradually improve its accuracy over time. Some of the benefits to this intelligence include automation, improved efficiencies, predictive analytics and better accuracy.
Considering the way that AI and machine learning continues to shape the global technology landscape, AI Magazine explores some of the current leading machine learning innovations.
10. Generative Adversarial Networks (GAN)
GANs refer to a class of machine learning frameworks and a prominent framework for approaching generative AI (Gen AI), pioneered by Research Scientist Ian Goodfellow. It works to train two neural networks to compete against each other to generate more authentic new data from a training dataset.
The technology is ultimately a way of producing stronger solutions for implementations like differentiating between different kinds of images. Some of the leaders in GAN discovery include the likes of Samsung Group and Alphabet.
9. TinyML
TinyML is a type of machine learning that allows models to run on smaller and less powerful devices. It involves hardware, algorithms and software that can analyse device sensor data with very low power consumption - thereby making it ideal for battery-operated devices.
Multiple industries can utilise Internet of Things (IoT) devices with TinyML algorithms to track and make predictions on collected data. Some of the benefits to running the TinyML program include reduced power consumption, low latency, user privacy and a reduced bandwidth.
8. Autonomous vehicles
Autonomous vehicles are capable of sensing its surrounding environment and can operate without the involvement of a person. Currently, society is most familiar with the autopilot function on car types such as Tesla, which acts as an extra assistant for the driver in certain situations.
Recently, Volkswagen announced that it would start to introduce ChatGPT as a voice assistant to some of its vehicles in 2024. We have previously reported that the decision expects to unlock a range of capabilities that extend past conventional voice control.
7. AI ethics
Subject to much discussion in the current technology landscape, AI ethics refers to a set of values and guidelines that are accepted standards of right and wrong to guide the safe development of AI technologies. Currently, there is no overall consensus over what these guidelines are, although world leaders and large businesses are working to set their own standards.
AI ethics are important, especially given anxieties over AI supporting or replacing human intelligence. In the business landscape, possible regulations would do well to support human workforce development.
6. Internet of Things (IoT) and machine learning
Using IoT technology with AI together aims to open up more unique opportunities. AI has the ability to enhance IoT’s capabilities by enabling data analysis, predictive insights, automaton and intelligent decision-making. AI can also compound the benefits of IoT by adding human-like awareness and decision-making to the environment.
AI then processes the data generated by IoT devices to make IoT systems smarter and more efficient. Some clear examples of AI and IoT working together are self-driving cars, collaborative robots and drones, to name a few.
5. Deep learning
Deep learning is a method of AI that teaches computers to process data much like the human brain does. Deep learning models can recognise complex patterns in pictures, text, sounds and other data to produce accurate insights and predictions.
Plenty of companies use deep learning in order to harness AI, including the likes of Google DeepMind, OpenAI and IBM. Deep learning is used in plenty of image recognition tools, natural language processing (NLP) and speech recognition software and thrives in more complex environments.
4. Improved cybersecurity
Cybersecurity is a key industry that uses machine learning, with AI applications able to identify cyber threats, combat cybercrime and enhance current antivirus software. Whilst there is concern that AI could negatively impact the cybersecurity landscape if used for exploitative purposes.
However, there are plenty of ways that AI can be harnessed ethically and responsibly in a cybersecurity capacity. Businesses must ensure that they are making strategic and sensible decisions to adapt towards positive AI, so as to best protect their company.
3. No-code AI
By taking advantage of no-code machine learning, developers can now readily access machine learning applications. It allows for automation via plug-and-play or drag-and-drop, leveraging machine learning to allow users with no AI coding skills to optimise daily operations and solve complex business problems.
With codeless machine learning, developing a system software no longer requires an expert. This could be a great advantage for smaller businesses that do not have the available funds to maintain an in-house team of scientists, for example.
Some of the best examples of no-code AI include Amazon SageMaker, DataRobot and Google AutoML.
2. Machine learning operationalisation management (MLOps)
MLOps focuses on the practice of developing machine learning software solutions that focus on efficiency and reliability. The primary purpose of MLOps is to streamline the development process of machine learning solutions so that they are of greater value to a business.
Using these applications allow organisations to utilise DevOps principles for machine learning and task automation. MLOps does this by providing a new formula for combining machine learning systems deployment and development into one method. MLOps also helps deal with business challenges, including communication, scalability and management of sensitive data at scale.
1. Hyperautomation
Automation has fast become central to AI and machine learning technology, in line with business digital transformations. When it comes to their operations, businesses now want increased speed and accuracy.
Hyperautomation refers to the automation of many company processes. It involves combining a wide variety of tools, such as robotic process automation (RPS) and AI to improve business decisions, efficiency and safety throughout the business. One of the many benefits of hyperautomation is that it comes in a wide variety of platforms and can also be implemented on top of the technologies that companies already have.
******
Make sure you check out the latest edition of AI Magazine and also sign up to our global conference series - Tech & AI LIVE 2024
******
AI Magazine is a BizClik brand